We love solving hard problems from evolution and adaptive immunology.
We enjoy all facets of computational biology research, from diving deeply into biological questions, to mathematical and statistical analysis, algorithm development, and efficient algorithm implementation.
Bayesian phylogenetics via optimization
Phylogenetic (evolutionary tree) inference is important for genomic epidemiology and for our understanding of evolution. Bayesian phylogenetic analysis allows us to assess and integrate out tree estimate uncertainty to obtain more reliable estimates of other model variables of interest (e.g. transmission rates). Current Bayesian methods rely on Markov Chain Monte Carlo, which does not scale to large data sets. We are developing an alternative formulation in which we perform systematic search to find a structure that represents the collection of trees in the high-posterior-density region, paired with variational inference for continuous parameters on this structure.
Phylodynamics with many sequences
With SARS-CoV-2 we are in a completely new “big data” regime at the intersection of phylogenetics, immunology, and epidemiology. This big data stresses existing inferential tools to the breaking point, yet offers many new opportunities to start afresh. We are developing new frameworks for inference in this regime.
Foundations of phylogenetics
Phylogenetic trees are complex objects of inference, with intertwined discrete (tree structure) and continuous (dates, rates) structure. Inference leads to very interesting challenges in statistics and discrete math. We cannot resist interesting problems on the essential elements of phylogenetic inference.
Antibody sequence analysis
B cells, the cells that make antibodies, develop according to a beautiful and complex evolutionary process. One can sequence the result of this process in high throughput, but learning the evolutionary history of these sequences requires carefully-designed algorithms. We develop these algorithms and apply them in collaborative projects to learn about how antibodies develop in response to specific pathogens such as HIV.
Learning mechanism and immune history from immune receptors
The adaptive immune system “learns” to recognize pathogens through highly diverse immune receptors called B and T cell receptors. The DNA sequences of large collections of these receptors can now be readily obtained using high-throughput sequencing. We develop methods to learn about immune history, genetics, and immunological mechanism using these sequences: these are additional topics compared to the evolutionary questions considered just above.
Immunology on the protein level
The outcome of an immune response depends on the fine details of protein-protein interactions. These interactions can be probed in high-throughput assays called “deep mutational scans.” In collaborative work, we develop means of learning from these rich data, and develop models to predict future interactions in the face of evolutionary change.
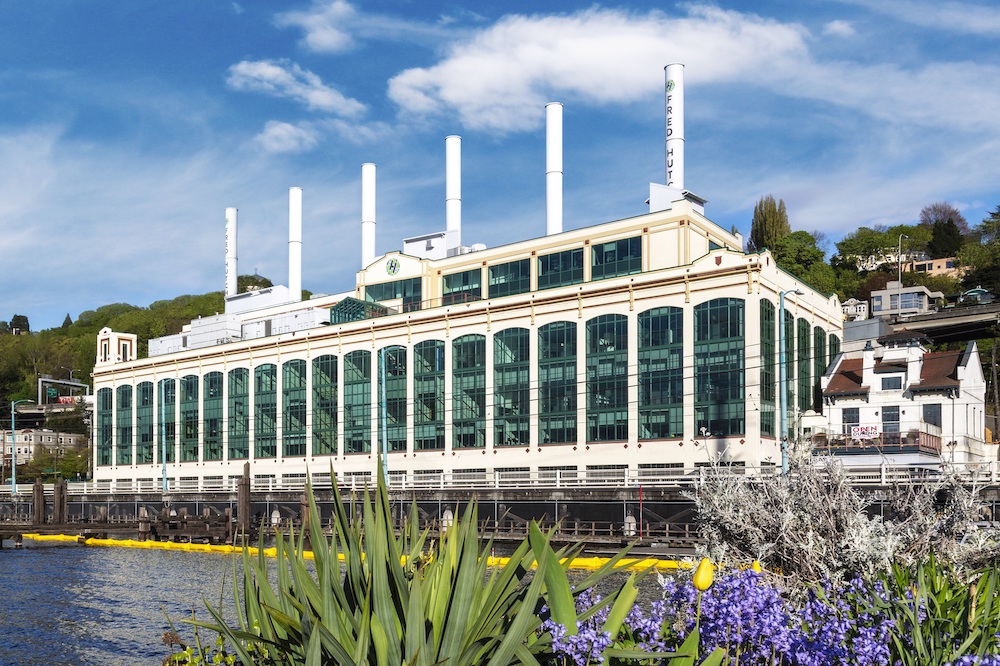
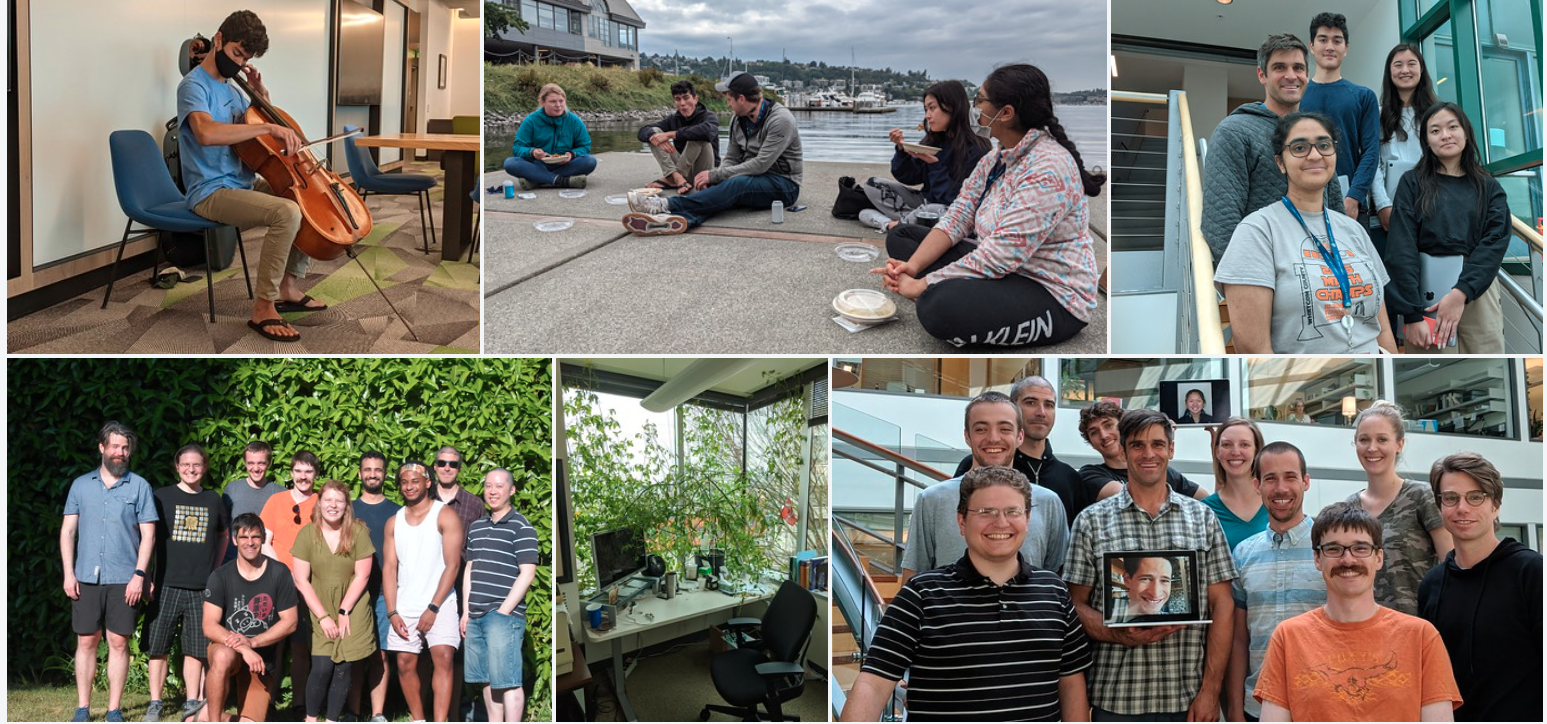
Thank you to taxpayers and private foundations for supporting our work.